Note
Go to the end to download the full example code.
Advection-Diffusion Equation#
Here we use the discretize package to model the advection-diffusion equation. The goal of this tutorial is to demonstrate:
How to solve time-dependent PDEs
How to apply Neumann boundary conditions
Strategies for applying finite volume to 2nd order PDEs
Derivation#
If we assume the fluid is incompressible (
where
where
We then apply the weak formulation; that is, we take the inner product of each equation with an appropriate test function.
Expression 1:
Let
The source term is a volume integral containing the Dirac delta function, thus:
where
where
Expression 2:
Let
If we use the identity
If we assume that
where
Expression 3:
Let
By evaluating the inner products according to the finite volume approach we obtain:
where
Final Numerical System:
By combining the set of discrete expressions and letting
Since the Neumann boundary condition is being used for the variable
where
For the example, we will discretize in time using backward Euler. This results
in the following system which must be solve at every time step
Import Packages#
Here we import the packages required for this tutorial.
from discretize import TensorMesh
from scipy.sparse.linalg import splu
import matplotlib.pyplot as plt
import matplotlib as mpl
import numpy as np
from discretize.utils import sdiag, mkvc
Solving the Problem#
# Create a tensor mesh
h = np.ones(75)
mesh = TensorMesh([h, h], "CC")
# Define a divergence free vector field on faces
faces_x = mesh.gridFx
faces_y = mesh.gridFy
r_x = np.sqrt(np.sum(faces_x**2, axis=1))
r_y = np.sqrt(np.sum(faces_y**2, axis=1))
ux = 0.5 * (-faces_x[:, 1] / r_x) * (1 + np.tanh(0.15 * (28.0 - r_x)))
uy = 0.5 * (faces_y[:, 0] / r_y) * (1 + np.tanh(0.15 * (28.0 - r_y)))
u = 10.0 * np.r_[ux, uy] # Maximum velocity is 10 m/s
# Define vector q where s0 = 1 in our analytic source term
xycc = mesh.gridCC
k = (xycc[:, 0] == 0) & (xycc[:, 1] == -15) # source at (0, -15)
q = np.zeros(mesh.nC)
q[k] = 1
# Define diffusivity within each cell
a = mkvc(8.0 * np.ones(mesh.nC))
# Define the matrix M
Afc = mesh.dim * mesh.aveF2CC # modified averaging operator to sum dot product
Mf_inv = mesh.get_face_inner_product(invert_matrix=True)
Mc = sdiag(mesh.cell_volumes)
Mc_inv = sdiag(1 / mesh.cell_volumes)
Mf_alpha_inv = mesh.get_face_inner_product(a, invert_model=True, invert_matrix=True)
mesh.set_cell_gradient_BC(["neumann", "neumann"]) # Set Neumann BC
G = mesh.cell_gradient
D = mesh.face_divergence
M = -D * Mf_alpha_inv * G * Mc + Afc * sdiag(u) * Mf_inv * G * Mc
# Set time stepping, initial conditions and final matricies
dt = 0.02 # Step width
p = np.zeros(mesh.nC) # Initial conditions p(t=0)=0
I = sdiag(np.ones(mesh.nC)) # Identity matrix
B = I + dt * M
s = Mc_inv * q
Binv = splu(B)
# Plot the vector field
fig = plt.figure(figsize=(15, 15))
ax = 9 * [None]
ax[0] = fig.add_subplot(332)
mesh.plot_image(
u,
ax=ax[0],
v_type="F",
view="vec",
stream_opts={"color": "w", "density": 1.0},
clim=[0.0, 10.0],
)
ax[0].set_title("Divergence free vector field")
ax[1] = fig.add_subplot(333)
ax[1].set_aspect(10, anchor="W")
cbar = mpl.colorbar.ColorbarBase(ax[1], orientation="vertical")
cbar.set_label("Velocity (m/s)", rotation=270, labelpad=5)
# Perform backward Euler and plot
n = 3
for ii in range(300):
p = Binv.solve(p + s)
if ii + 1 in (1, 25, 50, 100, 200, 300):
ax[n] = fig.add_subplot(3, 3, n + 1)
mesh.plot_image(p, v_type="CC", ax=ax[n], pcolor_opts={"cmap": "gist_heat_r"})
title_str = "p at t = " + str((ii + 1) * dt) + " s"
ax[n].set_title(title_str)
n = n + 1
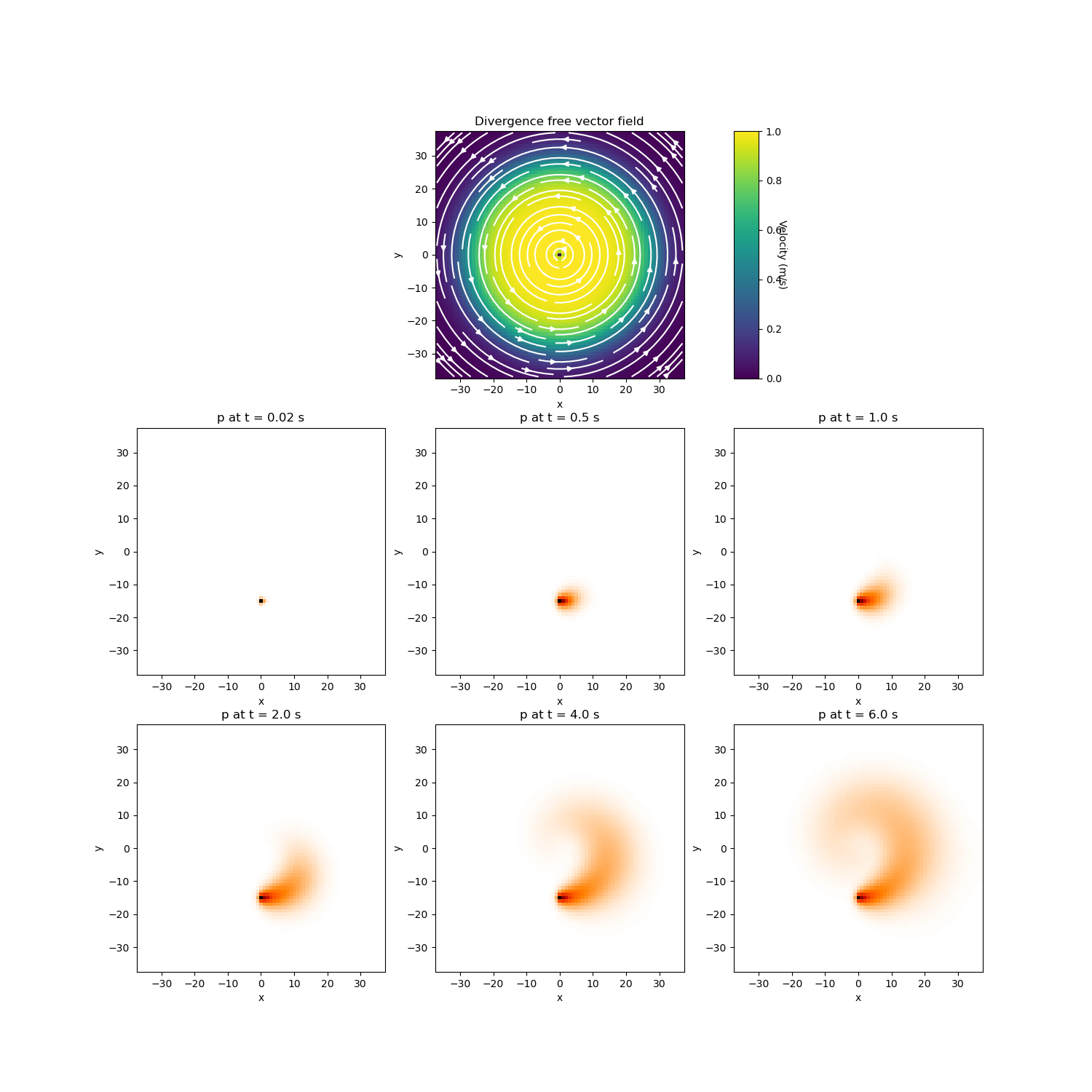
/home/vsts/work/1/s/tutorials/pde/2_advection_diffusion.py:227: SparseEfficiencyWarning: splu converted its input to CSC format
Binv = splu(B)
Total running time of the script: (0 minutes 0.942 seconds)